Semantic search represents a major upgrade from traditional keyword searching. It uses NLP and machine learning to grasp user intent, not just match words. The technology interprets relationships between concepts, building knowledge graphs that connect related information. For users, this means more relevant results even with imperfect queries. E-commerce platforms particularly benefit from this smarter approach. The technology continues evolving, with systems getting increasingly adept at understanding our messy human communication.
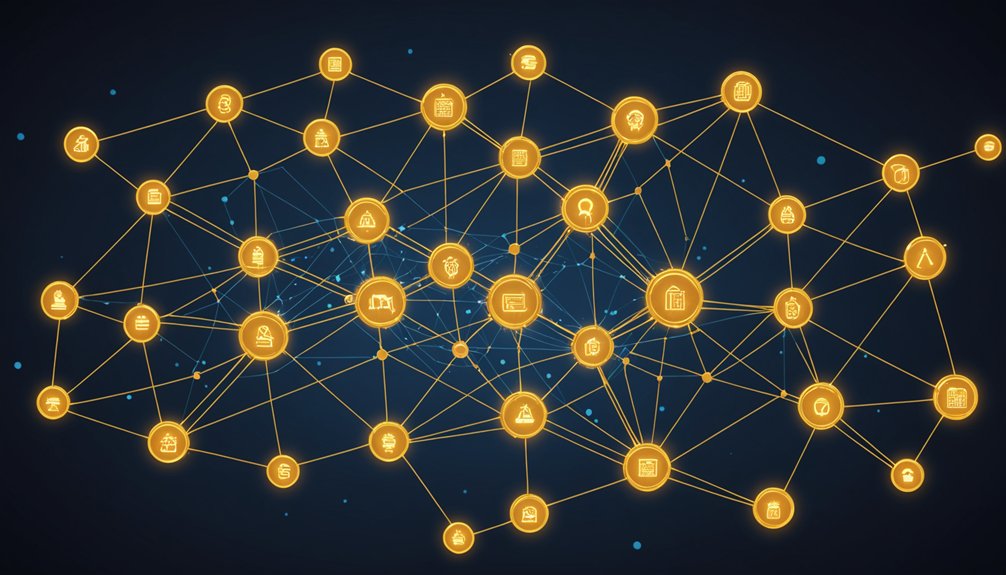
While traditional search engines once ruled the digital landscape with simple keyword matching, semantic search has changed the game forever. Those days of typing exact phrases and hoping for the best? Gone. Semantic search digs deeper. It actually understands what users want, not just what they type. Pretty revolutionary stuff.
Semantic search leverages Natural Language Processing and Machine Learning to interpret the relationships between words. It's not rocket science, but it's close. The technology analyzes context, figures out intent, and delivers results that actually make sense. Like chain of thought processing, it breaks down complex queries into logical steps to better understand user intent. Much like deep tech innovations in AI and quantum computing, it represents a fundamental shift in how machines process information. Imagine that! A search engine that understands you're looking for coffee shops when you type "places to get caffeine nearby." No more endless scrolling through irrelevant garbage.
Gone are the days of exact-match searching. Semantic search gets what you mean, not just what you type.
The evolution from keyword matching to semantic search wasn't overnight. It took years. But the transformation has been worth it. Users get better results. Period. The system learns from every interaction, every click, every query. Machine learning algorithms adjust and improve constantly. They're never satisfied, always getting smarter.
Knowledge graphs are the secret weapon of semantic search. They connect concepts, creating webs of related information. Think of them as the brain behind the operation. When you search for "apple," the system knows to ask: Fruit or tech company? Context matters.
In today's digital chaos, semantic search isn't just nice to have—it's essential. Users demand relevance. They expect systems to understand them. And why shouldn't they? The technology exists. It works. It delivers. Semantic search significantly enhances information discovery by mapping queries to synonyms or related terms, creating a more intuitive search experience.
NLP helps parse through language nuances, catching synonyms, different phrasings of the same question, even slang. It's like having a linguist working behind the scenes of every search. Semantic search doesn't just find information; it understands it. And in a world drowning in data, understanding is everything. E-commerce platforms particularly benefit from these capabilities, enhancing product recommendations based on contextual understanding of customer searches.
Frequently Asked Questions
How Does Semantic Search Impact SEO Strategies?
Semantic search has revolutionized SEO. Gone are the days of keyword stuffing. Now, it's about context, user intent, and quality content. Companies must adapt or die.
Structured data? Essential. Topic clusters? Non-negotiable. The focus has shifted to natural language, thorough answers, and contextual relevance.
Voice search is changing the game too. SEO professionals can't just count keywords anymore. They need to understand human behavior. It's a whole new ballgame.
Can Semantic Search Work Across Multiple Languages?
Yes, semantic search absolutely works across languages.
Modern systems leverage multilingual embedding models that understand context beyond words. Meilisearch, OpenSearch, and Cohere offer tools that automatically detect language and deliver relevant results regardless of the user's native tongue.
It's not perfect though. Cultural nuances still trip up algorithms sometimes.
For global businesses, this technology creates huge advantages—reaching wider audiences while maintaining relevance.
Pretty impressive stuff, actually.
What Computational Resources Are Required for Implementing Semantic Search?
Implementing semantic search is no small feat. It demands serious computational muscle—high processing power for complex vector calculations and hefty memory to handle dimensional spaces.
Storage requirements? Massive. Organizations need substantial data capacity for datasets and knowledge graphs.
Network bandwidth matters too, especially for cloud setups. The resource intensity makes cost a real concern. Small teams often struggle with scalability.
That's the reality—semantic search eats resources for breakfast.
How Is User Privacy Maintained in Semantic Search Systems?
User privacy in semantic search relies on multiple safeguards.
Systems implement data access controls based on user identity, preventing unauthorized snooping. Data stays within the user's designated tenant—right where it belongs.
Privacy-enhancing technologies like anonymization and differential privacy add extra protection. Companies must comply with GDPR and similar regulations, obviously.
The best systems give users control over their data and maintain transparent policies. No secrets, no surprises.
What Metrics Evaluate Semantic Search Accuracy Versus Traditional Search?
Semantic search accuracy versus traditional search is evaluated through multiple metrics.
Precision measures relevant results returned. Recall tracks completeness. F1 score balances both. Semantic similarity assesses intent matching quality. User engagement metrics like click-through rates reveal satisfaction.
Traditional search just counts keywords. Semantic search? It understands context, handles complex queries better, and recognizes entities. Machine learning keeps improving it.
The difference? Night and day.